Why to validate AI/ML GxP systems?
Providing an acceptable level of credibility for data-driven algorithms is of paramount importance due to the inherent low transparency of the technology. Hence, in order to guarantee an adequate level of quality mitigating the high risk to produce incorrect or unreliable results, regulatory agencies such as the US Food and Drug Administration (FDA) and the European Medicines Agency (EMA) have established high-level requirements in order to ensure safety and effectiveness of AI-based products and services.
The requirements mentioned above are mostly related to:
- Data quality (i.e., the quality of the data used to train and test the AI and ML models; the data must be representative of real-world conditions and must be free of errors and biases);
- Algorithmic stability (i.e., to guarantee that AI and ML models are stable and do not produce significantly different results when trained or tested on different datasets);
- Model performance (an evaluation of the performance of the AI and ML models must be performed to ensure that they are accurate and reliable);
- Explainability (i.e., to understand and document the logic that leads the decision-making process of AI and ML);
- Documentation and record-keeping (i.e., maintaining updated records and documentation related to the validation).
To satisfy these requirements, a Validation process is required, demonstrating compliance with regulatory requirements and maintaining the integrity and accuracy of any AI or ML model over time.
About the validation of AI/ML systems
Based on the most recent regulations which have been released about AI/ML Validation, the Advanced Technologies Competence Center of PQE Group developed an effective risk-based strategy to reach the required level of compliance:
- ASME V&V 40-2018
- GAMP 5v2
- ISO_IEC_38507_2022(en) - Governance of IT - AI implications
- ISO_IEC_TS_4213_2022(en) - Assessment of ML classifier performance
Integrating the content of the above-mentioned guidelines with the new Computer Software Assurance (CSA) approach and with Agile Development methodology, PQE group experts have developed a dedicated framework to guarantee an acceptable level of credibility of the algorithms to be validated.
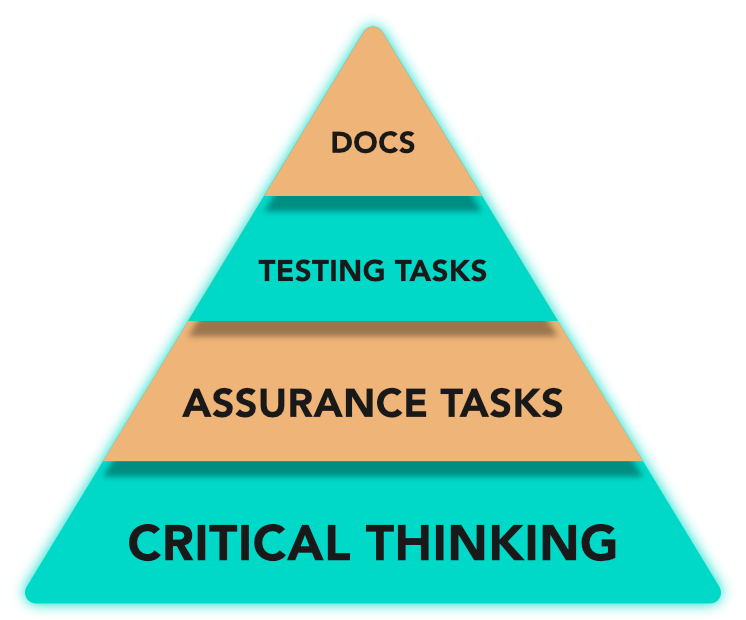
Figure 1 CSA Approach scheme
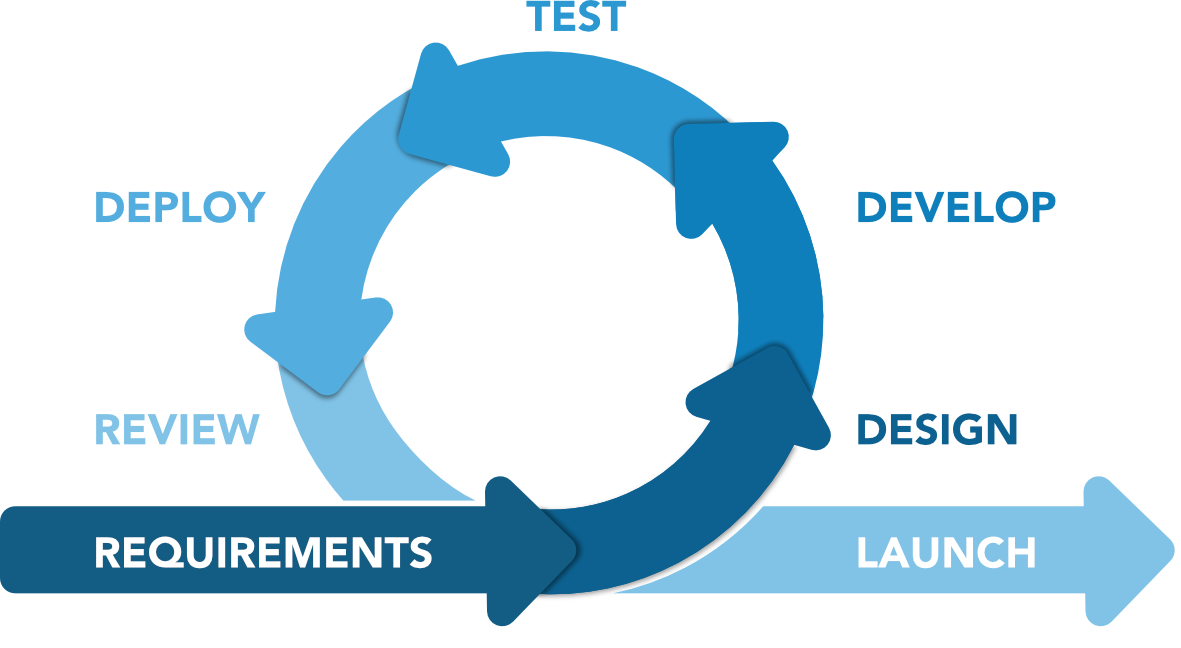
Figure 2 Agile methodology
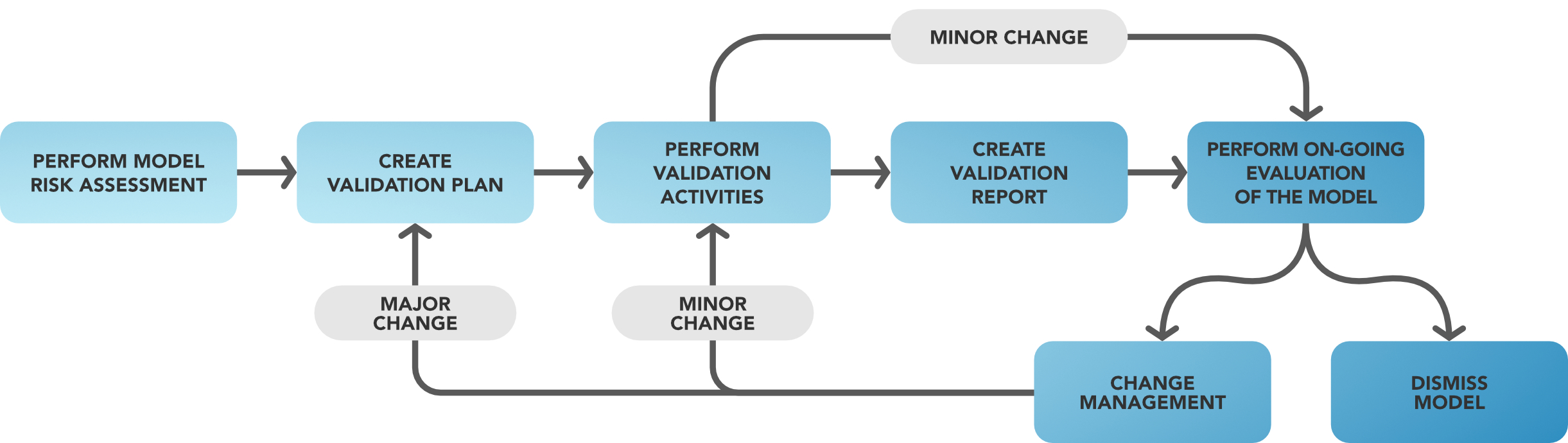
Figure 3 Model Validation Lifecycle
The Validation Activities to be carried out on an AI/ML Model shall always include the following activities:
- Performing the Model Risk Assessment;
- Creating the Validation Plan;
- Performing the Validation Activities including testing;
- Creating the Validation Report;
- Applying the Change Management;
- Performing Ongoing Evaluations to maintain the validated status of the model, until it is decided to dismiss it.
As already mentioned, the Validation process needs also to rely on the CSA approach in order to reduce the foreseen effort, giving more space to the critical thinking phase and assurance tasks, optimizing testing activities and reducing documentation.
In particular, the core of the process is the determination of the risk. This leads to achieve balance in validation activities, guaranteeing a proper regulatory acceptance level and a reasonable amount of documentation to be created and maintained.
In parallel, a data validation process is needed in order to fulfill all Data Integrity and data quality requirements.
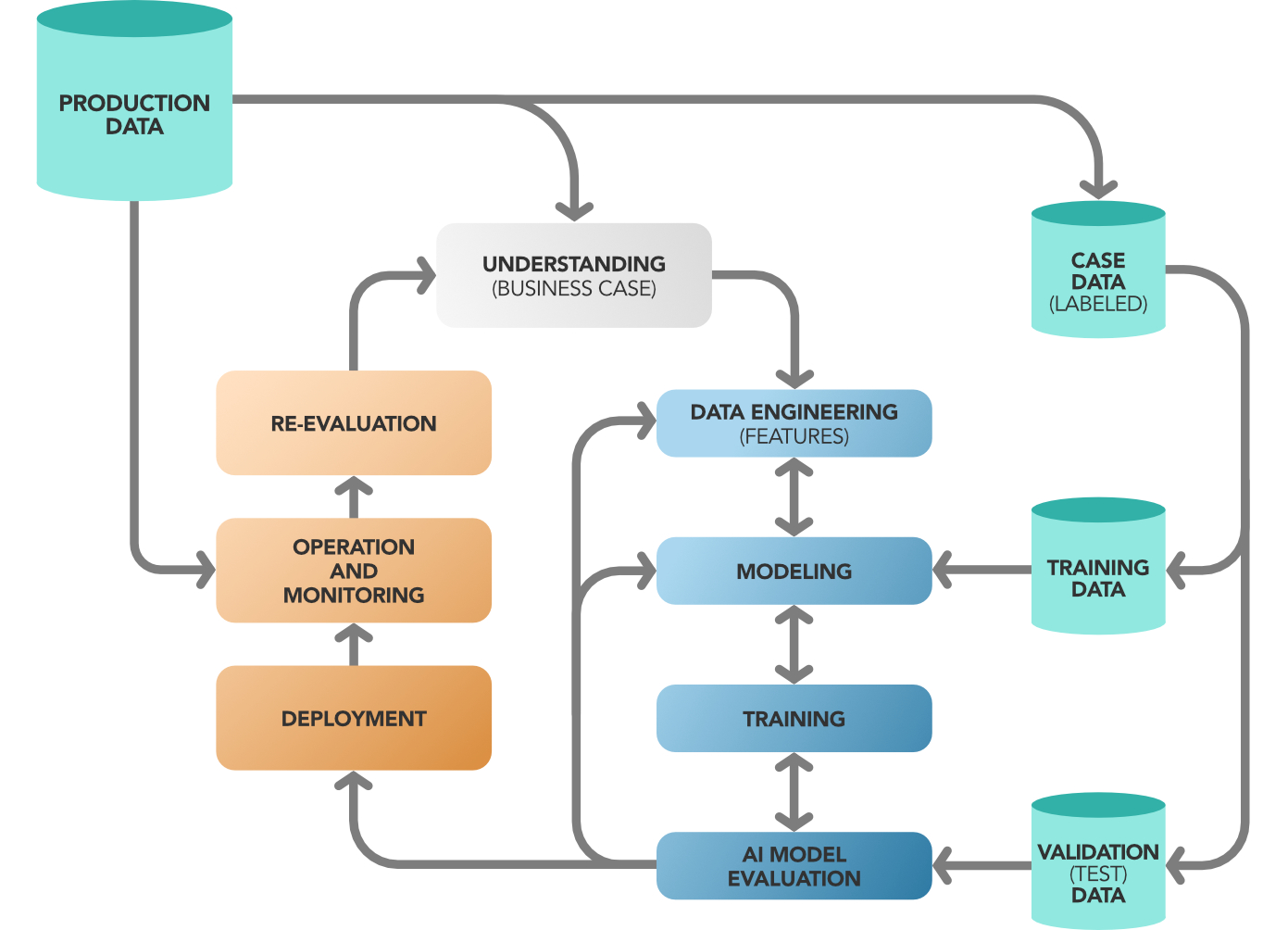
Figure 4 Data lifecycle and validation integrated with Model maintenance lifecycle
In particular, it is not possible to validate an AI/ML algorithm without taking care of the format of the data and of the data engineering process in general.
Conclusions
In summary, the validation of GxP systems encompassing AI/ML models/algorithms is a critical step in ensuring the quality and safety of regulated products. It is essential to consider factors such as data quality, algorithmic stability, model performance, model explainability, and documentation in order to ensure the reliability and effectiveness of these models.
The PQE Advanced Technologies team developed a solid strategy to reach compliance, combining main validation milestones with CSA and Agile Methodologies principles. This will optimize the activities, balancing the amount of documentation to be produced and getting from the other side the proper level of regulatory acceptance, also keeping in consideration data cleaning and data engineering workflows, which strongly impact the model efficacy and maintenance.